Artificial Intelligence and Machine Learning as added value for your business
Added Value through Artificial Intelligence
Wording and overview: How to find your way through the buzzword jungle
Artificial Intelligence (AI)
The term "artificial intelligence" is a generic term for all methods that attempt to imitate partial aspects of human intelligence by imitation. In this context learning ability, perception, logical decision making and pattern recognition play a significant role. For example systems can recognize animals on pictures without knowing what an animal is, how it lives, moves etc.. But if a task goes beyond the learned special discipline, artificial intelligence fails. Rule-based "expert systems", such as chess computers, can also be understood as a form of artificial intelligence.
Machine Learning (ML)
"Machine learning" refers to a procedure in which artificial intelligence is represented by a computer system that can learn complex patterns and structures from data. Statistical models and algorithms are able to learn a relationship between input and output data without human intervention and also transfer this relationship to new data. The dynamic algorithm of a machine learning model tries to optimize a user defined target function. This distinguishes machine learning models from classical decision systems with rigid decision rules.
Deep Learning (DL)
Deep learning is a much considered sub-area of machine learning, which is inspired by the structure and functioning of the human brain. In deep learning, modelled neural networks process information. Data is fed into the network as input and in deeper layers of the network (deep) more and more complex structures can be learned (learning) to produce certain results. Such neural networks are able to learn from historical data fed by modern algorithms. Based on the output, forecasts, decisions or patterns can be derived.
How can Artificial Intelligence and Machine Learning enrich your business?
Forecasts and predictive analytics for decision-making processes
Machine learning algorithms are able to recognize relationships and trends in huge amounts of data and from this derive forecasts and recommendations for important sales and cost-relevant business decisions. In this way, the company's profit can be directly increased by AI.
Clustering and Data Mining
A further use case of machine learning is to recognize structures, dependencies and trends in large unstructured data sets. For example, it is possible to better structure data, make it understandable and generate business-relevant insights from it.
Introduction to Machine Learning:
Success factors and pitfalls in a Machine Learning Project
With an incredible range of applications and rapidly developing frameworks, machine learning is a...
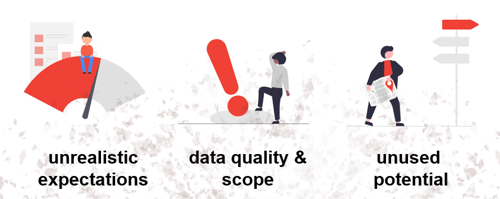
Machine Learning use cases for companies
Machine Learning (ML) will continue to be a popular answer to current business challenges in 2021....
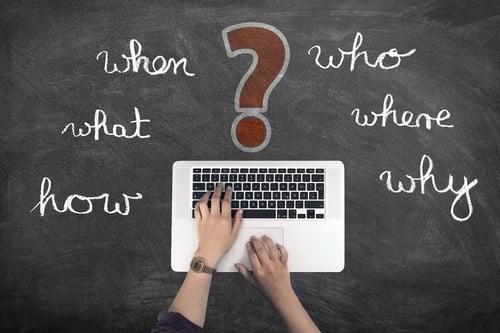
Exemplary AI Use Cases in different business areas
Nearly all business areas and industries can benefit from the use of artificial intelligence.
Find out how and in which areas artificial intelligence is already being used successfully today:
AI in Finance
Cash-In Prediction
Open customer invoices are often not paid on the due date. With machine learning, late or premature payments can be better predicted, enabling intelligent liquidity and cash flow planning.
AI in HR
employee fluctuation
AI makes it possible to predict the fluctuation probability of an employee. In this way, better decisions can be made in HR and the recruitment needs of different company divisions can be better planned.
AI in Procurement
Estimate demand and costs
In procurement, machine learning helps to calculate the actual total costs from the planned material, personnel and other costs. Possible cost increases can be identified and suitable countermeasures taken.
AI in Sales
Revenue Forecast
On the basis of previous leads and open negotiations, the actual turnover can be predicted.
Using Machine Learning in business
Planning with Machine Learning - Cash-In Prediction
A reliable forecast of incoming payments is crucial for the planning of cash and cash equivalents. If it is to be predicted when invoices will be paid, a complex framework of internal and external factors plays a role, which are optimally evaluated with machine learning. Calendar-based information such as weekdays and public holidays, key figures about the payment behavior of a debtor and current global conditions are obtained from internal and external data sources. Daily forecasts are then automatically generated and forwarded to the responsible parties in the form of reports. There, the information helps to estimate the short-term capital requirement or surplus.
Sales with Machine Learning - Order Conversion Probability
Sales can also be more efficiently aligned through the use of machine learning. Based on historical business data about a customer and various key figures of an offer, a forecast can be made about the probability of success of an offer. This makes it possible to compare possible offer alternatives and to select the best one.
Automation with Machine Learning - Automated Invoice Processing
Automated invoice processing must be able to handle both paper invoices and invoices in digital formats (email, PDF). For this purpose, optical character recognition (OCR) is used to process scanned and photographed documents. Data extraction and validation provides the required text elements, which are transferred to a database. A subsequent classification of the invoices and conditional further processing can also be initiated by machine learning. This process saves time, which results in reduced processing costs.
Detecting Fraud with Machine Learning - Fraud Detection
Digital business processes in particular are exposed to an increased risk of attack and fraud. machine learning supported fraud analysis can help to increase security not only in purchasing. Instead of processing all types of fraud individually, the system issues a warning in case of behavioral deviations of customers or irregularities in transactions. The particular challenge is to create a system that reliably detects fraud without triggering an unnecessary flood of warnings that would rather take the strain off the employees than relieve them.
Optimize with Machine Learning - Customer Segmentation
Target group-oriented or behavior-based customer segmentation - there are many ways to boost marketing and sales. With a customer segmentation, those customers are identified who will stimulate your business. In addition to the profitable ones, there are also customers who are comparatively unprofitable or not profitable at all due to low sales. In both cases, customer acquisition and retention causes costs through discounts, support and marketing campaigns. Invest your capital optimally in the customers with the highest profitability. With machine learning and the RFM (Recency, Frequency, Monetary) classification approach, an individual indicator per customer can be created based on sales and order interval, among other things, which can optimize further sales activities. In this context, the data-driven detection of customer churn can also be implemented.
Find interesting articles about this topic in our Blog
Apache Airflow 3.0: Everything you need to know about the new release
Apache Airflow 3.0 has been publicly available since April 22, 2025. It is the first major release...
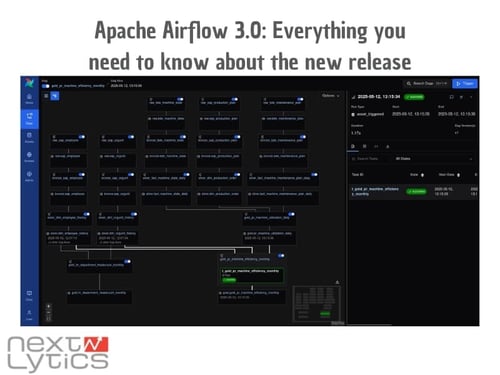
Databricks integration in SAP Business Data Cloud: Interview with M. Ingerfeld
In February 2025, SAP and Databricks finally announced tangible results from their 2-year-long...
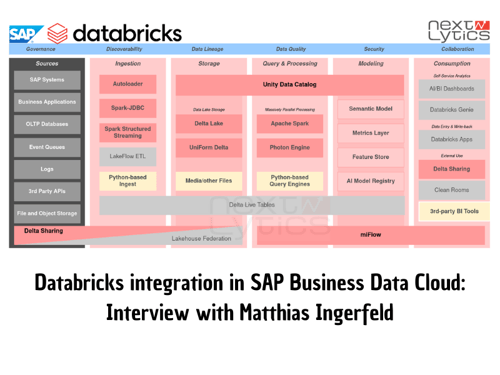
Business Data Cloud - What is it all about?
Yesterday we saw SAP present their long awaited announcement at the SAP Business Unleashed 2025...
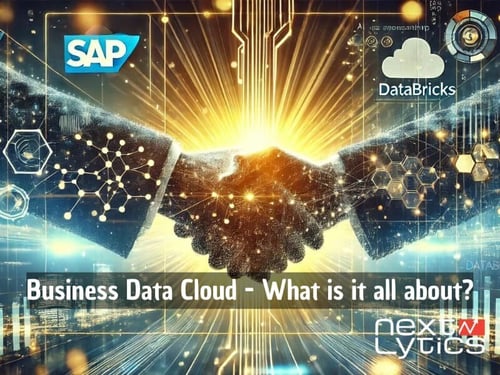