The world will be different after the coronavirus pandemic, most experts are sure of that much, but what does this mean for their forecasts and planning tools? You don't have to be a fortune teller to predict that the crisis will shift market shares and that customer behavior patterns will change significantly.
In the airline industry alone, bankruptcies and consolidations mean that the industry will undergo massive change. Added to this is the fact that the crisis has made video conferencing, which has become the standard, a trend towards significantly fewer business trips in the lockdown phase. This form of change will also be observed in other areas and will have a long-term impact on industries such as the automotive sector, tourism and trade. But what does this mean for your machine learning models? Can patterns from historical data still be reliable indicators for anticipating future developments? Or should we go back to archaic tools like the popular Excel sheet to get through the next planning cycle?
The answers to this depend on several factors:
- What impact do you expect the crisis to have on your business model?
- How much is model-based planning accepted in your company?
- Do you have data science competence in-house?
- What tools are available to you?
Let us examine the individual factors in more detail step by step and ask ourselves what we can learn and adapt from the epidemiologists' approach to assessing the coronavirus crisis.
What Impact do you expect the crisis to have on your Business Model?
The novel coronavirus pandemic and the resulting lockdown can be understood as a special business event that will have an impact on the short-term but also long-term growth trend of the company. There are three phases that need to be considered for assessment and development:
(1) The state of the business before the crisis occurred
(2) The impulse as a direct result of the event
(3) The lasting effect after the end of the crisis
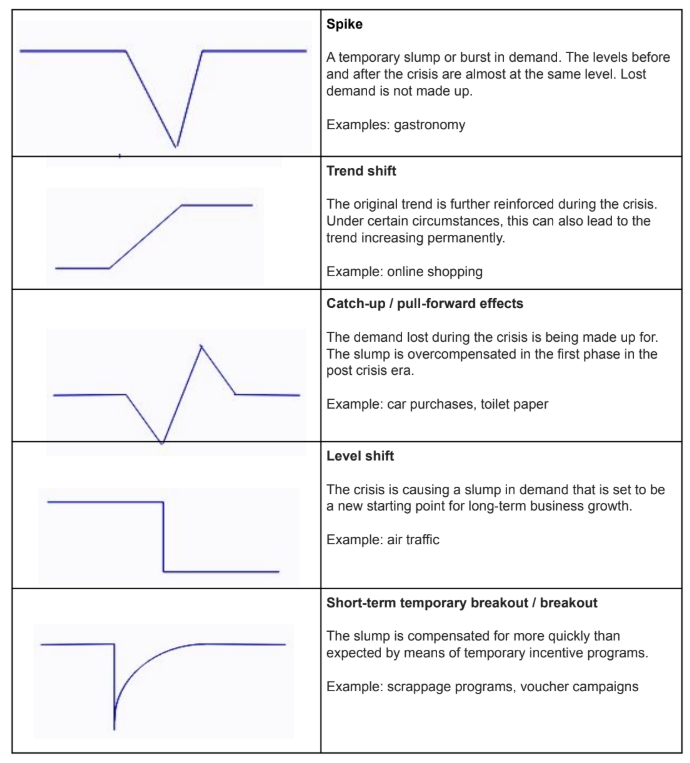
In case of a longer-lasting crisis, it is also entirely possible for combinations of the impulse variants mentioned to occur or even be overlaid during course of coping with this disaster. The individual opinions of your experts and managers are essential for an assessment of which variant is to be expected for your company throughout the course of the ongoing crisis. Forecast models that are based exclusively on historical data, especially in times of a unique historical event such as the current pandemic, cannot correctly anticipate future developments.
Are machine learning methods not usable at all and are they now showing their dependence on historical data and thus their vulnerability to predict the future under changed conditions?
The answer is no, they are not superfluous, but right now the models need to be enriched and adjusted with empirical knowledge from the business units, which leads us to the next point.
How to bring SAP BW and state of the art Machine Learning together
How much is Model Based planning accepted in your company?
Above all, the current situation demands quick and decisive action with a very blurred view. In this emergency, quite a few businesses have resorted to trying and testing tools such as the Excel sheet, with which they can use their expertise of spreadsheets to the fullest extent. Often you fall back into old planning methods and obsolete techniques, which may deliver quick and easy-to-understand results, but do not necessarily have to be correct. Complicated dependencies in particular can no longer be modeled in this way and usually result in fallacies and apparent reductions in complex relationships, which could result in misinterpretations, particularly when simulating various future scenarios.
Machine learning models (ML models) do not have the reputation of being particularly transparent and are therefore often referred to as “black box models”. In fact, the price of ML models that are able to recognize complex structures in data is often a difficult to impossible interpretation of the recognized pattern. Techniques such as LIME, Shapley Values or Feature Importance are trying to shed some light on the results, but the results of these processes are usually cryptic and not particularly intuitive for a manager or user from the business unit. The interaction of business units and data science teams in the creation, interpretation and application of these models in daily business is all the more crucial. Companies with a more anchored mindset of data-driven decision support will find it much easier to accept model-based solutions and continue to see them as a key element in crisis management.
Perhaps the crisis will show which companies are more likely to have established such a culture and to deploy efficient teams of data and technical experts to deal with the crisis in a targeted manner.
Do you have data science competence in house?
If your own skills have already been built in-house, then hats off to you! You are in the great position of being able to get started and become active. Bring your data and technical experts together and set up analysis, forecasting and planning task forces in order to achieve the best possible results based on the interdisciplinary skills. The knowledge from existing models and the expected business development must be coupled with one another in order to enable a stable and reliable statement about future scenarios.
If your own competencies are not available in-house, the inclusion of an external partner would be advisable right now and the long-term development of in-house competencies in the area of data analysis and modeling.
What tools are available to you?
"If your only tool is a hammer, you will consider every problem a nail."
Mark Twain
One could describe the desperate clinging to archaic planning methods, which in their limited possibilities do not do justice to the necessary level of detail. Data analysis tools or platforms can be set up relatively quickly these days. Cloud solutions or sandbox variants make it much easier to get started and can sometimes be made available in just a few hours. And perhaps the momentum of times of crisis will make the introduction of new tools easier and will shorten the typical procedural errors through compliance, data protection and other internal institutions. If German schools and authorities are able to improvise digital lessons on short notice (if not fully and flawlessly), companies should be expected to do similar things.
The lack of suitable tools should not be an excuse, the lack of skills and experience can be compensated for by external partners.
By line of sight- What we can learn from epidemiologists
The political decisions within this crisis were largely made on the basis of factual and model-based findings. To do this it was necessary to build models that were composed of various influencing factors, but not all of them were known. A very simplified representation could therefore be:
Known knowledge + unknown knowledge + inexplicable effects = pandemic scenario
Known factors include all the elements that can be taken from existing data for this pandemic and that have an impact on the different scenarios. These are, for example, knowledge about the frequency of contact by different people, patterns of mobility, behavioral habits or seasonal effects. Unknown knowledge is understood to mean everything virus-specific, which is not sufficiently known or which may initially be adopted as a “best guess” from comparable virus infections. These are, for example, the coronavirus-specific attack rate, the reproductive number, lethality or the factor for asymptomatic diseases. In the early stage of the pandemic, many of these factors are unknown or not verified, which is why experts need to make an assumption based on their experience and dialogue with other experts. These estimates are then continuously compared with current data and readjusted. In this way, unknown factors can be calculated over time, which can then gradually be declared as known factors. In this phase, it is important to check and readjust the model parameters in short cycles.
Similarly, the challenge should now be with companies that should not simply rely on models from historical data alone, but they must incorporate the assessment from the experience of their experts more than ever into the models, while maintaining flexibility. For example, the shifts in market shares must be anticipated by experts or an estimate must be made about the changing customer behavior. In short iterations, these assumptions should be checked using current data and adjusted if necessary. How does this succeed for machine learning processes that learn complex relationships independently from historical data? Because it is not possible to intervene directly in the internal learning algorithms, there are two approaches to still incorporate assumptions by the experts in the model:
1. Adjusting the data
The training data is adapted to the desired effects. For example, market shifts can be adjusted to the future expected value using so-called sampling strategies (over- / undersampling) or level shifts can be encoded in advance. It is also possible to create new input features that incorporate an impulse as a new feature, but remains a difficult task for most variants.
2. Adjustment of the model results
The impulse variants or correction factors are applied to the result of the models and thus result in an adjusted final result. Techniques from signal processing, heuristics or time series analysis can combine impulse distributions with the model results and thus create a possibility to link systematics from the past with new assumptions.
In addition, changing the model variant, e.g. to classic regression models, always to be considered, as this may provide even more reliable results and more flexibility.
In Conclusion
Yes, what about the “New Normal” after Corona? That the glass ball has to be ground and polished is almost as certain as the Amen in the church. However, due to the urgency and complexity, do not fall back into old planning structures, but tackle the challenge with a task force consisting of data experts and continue to rely on the methods from the field of data science and machine learning as a basis for data-based planning and decision-making. Using various techniques, machine learning models can be expanded to include the knowledge of the experts in order to model assumptions and plan scenarios for the era after the pandemic.
If you are not yet completely convinced or you simply lack the necessary skills and tools, come to us, we would be overjoyed to help you prepare for the “New Normal”.
This article was published as part of the blog parade #TheAIFactory of the blog Ingenieurversteher.de
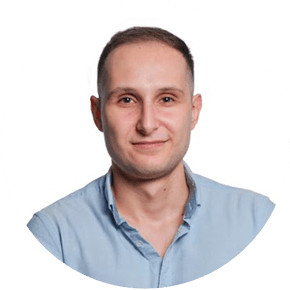