All your company‘s business units own processes around data they produce and use. They take pride and responsibility in providing high quality substracts to their colleagues from other departments. They have all the necessary tools and knowledge to implement changes to these data and their processing routines themselves. Measurable business value is drawn from your company‘s data in a cross-department collaborative effort that renders all participants sovereign actors with a clear understanding of purpose. Sounds too good to be true? Let‘s talk about the concept of data mesh.
Data mesh is one of the growing buzzwords in the business data community. The concept originates from the scientific observation of success factors and especially hurdles to successful implementation of company-wide data strategies, data and information distribution among stakeholders, and the underlying technical architecture and processes. In short: classic centralized data warehousing and the less strictly curated data lake approach introduce massive resource bottlenecks whenever changes need to be implemented.
These architectures designed by IT and data processing experts rely on that same technical expertise to provide central services to upstream producers and downstream consumers of data. Producers and consumers would usually be business units that should be responsible for their data and the insights they generate from them. Instead, they are structurally dependent on the ability of data warehousing or engineering teams to change the infrastructure and data output for them. As that resource runs short, formal service management is introduced, drawing a well-defined line of order and supply between departments to better distribute the available capacity. That further complicates things between what is ultimately just people who want to spend their time working on meaningful tasks.
A classic data warehousing architecture with centralized function-aligned teams for warehouse management and
data engineering may introduce bottlenecks in an organization’s data-driven value generation processes.
Business units are not in control of data they produce or consume.
How is the data mesh different? How could another technical architecture pattern alleviate any of these organizational challenges?
The data mesh is carried by four main principles:
- Data is treated as a product
- Decentralized domain teams own the data they produce and utilize
- All teams have access to a self-service infrastructure for data processing and storage
- Data governance is enforced by a federated computational model
Data mesh is thus obviously not just a technological approach but includes aspects of organizational structure, processes, and even company culture. From the beginning, it has been declared a “sociotechnical” approach, taking both social and technological processes within organizations into the equation. Better yet, there is no turnkey software you can simply buy, roll out, and have a successful data mesh implemented, but implementation is a highly customized individual process for every organization. The reason for this is the process-oriented nature of the definition of the data mesh architecture itself which can be achieved with any number of and combination of software solutions available depending on preferences of the stakeholders involved.
At its core, the data mesh approach is about the decentralization of responsibility as a means of scaling the infrastructure. At the same time, this elevates data product teams into a position of self-governing actor and advocate for the data they own and share with other teams. Ownership is given back to the people with actual expertise of the information encoded in data.
How to advance your business through
Artificial Intelligence and Machine Learning
The high ideals of data mesh come at the price of creating a strict and machine-actionable governance model. A meta data catalog is required that tracks all data products, who is responsible for them, how they are produced, what quality can be expected, who may access the data and who is in charge of granting access, how can data be retrieved, and finally which other data products depend on this item. These interconnections between data products create the name-giving mesh topology.
Social aspects of the transition into a data mesh architecture are not only the new responsibilities and roles within domain teams (usually business units, or groups within business units) and their interaction but also across teams. Governance and interdependencies of data products require resilient and scalable processes across all stakeholders in the mesh. As we already know from our democratic political system or agile project work methodology: high levels of freedom and self-governance require strict rules and responsibilities. It’s not a coincidence that “data democratization” and “data citizenship” are often referred to when discussing concepts and principles of the data mesh.
The data mesh approach aims to give ownership of data back to business departments where full understanding and
expertise in handling and interpretation of that data is originally located.
Newly assigned data product teams use self-service tools to create and curate their respective goods
and share them along value generating business processes.
The data mesh approach follows an overarching trend in technology towards responsible and self-organizing teams while introducing learnings and best practices from software engineering practice into a less explored domain. Fitting data products to well-defined metadata models and defining computational governance are translations of patterns well known from software dependency management. The separation of tasks and responsibilities into self-contained problems has already seen successful implementation in domain driven design, microservices architectures, and agile management frameworks. Data mesh is a logical evolution of how data management can be scaled beyond the sheer size of technological databases and data platforms.
Sounds great but like a lot of work? That’s why a data mesh journey starts with high level strategic questions about the goals and motivations and whether embarking on this ride is ultimately necessary. If your organization suffers from some of the initially outlined challenges and if after initial strategic discussions the decision is to move towards a data mesh architecture, the transformation is a gradual, iterative and incremental process. Creating the organizational and technical infrastructure to implement a data mesh is a process of constant learning and customization for the specific needs of your organizations’ realities. Many pieces of the puzzle are already spread out on the table, too, as some of our previous blog posts show: Decentralization is increasingly well-supported by platform software, be it SAP data warehouse solutions, all-in-one data science platforms, or highly specific platform components like self-service feature stores for machine learning developers. Data processing skills spread across business departments as data literacy is increasingly addressed and promoted by organizations, laying the foundation for decentralized data ownership.
We frequently experience that discussions about companies’ data strategy and data platform architecture touch on the topics of data democratization and challenges addressed by the data mesh approach. If the trend continues to grow in the same way as (software) engineering best practices and agile management methodologies have expanded into the fabric of organizations beyond the scope of just IT departments, the question is not if one should analyze the potential of adopting these concepts but when.
We hope this broad overview of the data mesh approach, its motivations and potential benefits has helped you get a better understanding of this trending topic. If you’d like to discuss your organizations’ specific needs and whether data mesh could be beneficial for you, we’re eager to learn about your challenges and happy to help finding and implementing optimal solutions.
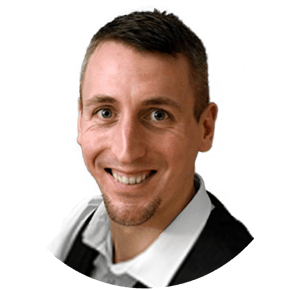