In a successful machine learning project, in addition to the famous data scientist, various roles with their own specialization must be filled. To help you keep track of who belongs to the machine learning team, we would like to present the roles and their areas of expertise in more detail in this article.
Domain Expert - Business Expert
As an expert for the actual use of the application, the Domain Expert brings in a great deal of knowledge around the project goals. He specifies the requirements and provides context for the data and results. With extensive knowledge of the processes and the underlying problem, he or she can assess the usefulness of the model. As core competencies, the Domain Expert should have specific department knowledge and an understanding of internal processes. Good communication skills and an interest in technical and statistical topics are also essential.
This enables him to contribute to the project in the best possible way. In so doing, he or she can inform the other team members about existing contexts and thus avoid redundant analyses. During the course of the project, he ensures that the machine learning solution is designed in accordance with requirements by providing ongoing feedback.
Data Engineer - Providing the Data
To ensure that the data ends up in the right place, quality and form, there is the project role of the Data Engineer. The Data Engineer provides the Data Scientist with the data for exploration. He or she is responsible for cleaning the data and administration of the databases and merging the different data sources. The essential part of the machine learning project deals with making the data available, which is why the number of data engineers is the first to grow as the project size increases.
Since its results shape the data model and thus flow directly into the model, subsequent changes and costly additions are a major reason for delays in the project. With changed features the complete model creation has to be repeated. This influence should not be underestimated even in an iterative model development.
For a suitable staffing, specialized knowledge in the area of databases and, if needed, Big Data should be available. The implementation of data merging and cleansing in terms of programming should also be covered by the competence profile.
How to advance your business through Artificial Intelligence and Machine Learning
Data Scientist - Development of the ML Model
Equipped with an intuitive understanding of data and excellent methodological skills, the Data Scientist starts the first draft of the model and thus the prototype. In the initial phase, the Data Scientist takes over the exploration of the data and thereby gains insights into which features are likely to be profitable for the model. Through skillful visualization, important relationships in the data are revealed. In the further course of the project, the Data Scientist serves as an all-rounder who supports the other machine learning team members. Close contact with the Domain Expert in particular is often very positive for the project result.
The competencies of a Data Scientist are a mixture of statistics and programming with a focus on typical Data Science frameworks, which can be open-source or proprietary.
Machine Learning Engineer - Making the Model productive
Making the final application available to its users is the job of the machine learning engineer. He or she optimizes the ML model and its performance and implements it in a productive system for sustainable benefit. When planning the system architecture, he or she draws on his or her wealth of experience and automates necessary process steps.
The ideal person for the role already has experience with the deployment and operation of machine learning applications and in particular brings special knowledge in the area of DevOps/ML Ops.
Of course, not all roles need to be covered by different people, but the areas of responsibility and expertise should be planned comprehensively in order to derive value from the machine learning model in a productive form. If the team is already in place and you are still looking around for suitable project ideas, feel free to take a look at our whitepaper.
Machine Learning Team Work
3 tips for assembling a successful machine learning team
Finally, we would like to make it easier for you to staff the project team with the following tips:
- Involve your business intelligence department
- Focus on going live in small projects
- The team does not stop with the members
If you need support in the planning and realization of machine learning projects, please do not hesitate to contact us. Our consultants have different areas of expertise and complement your machine learning team with the desired competencies.
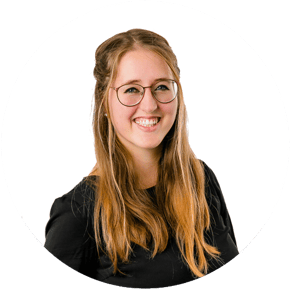